I currently research AI applied to finance and economics as well as teaching AI as part of my Criticality in Dynamical Systems postgraduate class. My earliest work in AI was implementing a shallow artificial neural network to simulate human-like perceptual expertise that mimicked the inferior temporal cortex of the human visual pathway. This led to developing a self-organising map of perceptual learning in the game of Go where 'expert' AIs could be compared with 'amateur' AIs, illustrating the different neural structures each AI had developed and what role each neuron played in perception, i.e. 'explainable AI'.
I currently research AI applied to finance and economics as well as teaching AI as part of my Criticality in Dynamical Systems postgraduate class. My earliest work in AI was implementing a shallow artificial neural network to simulate human-like perceptual expertise that mimicked the inferior temporal cortex of the human visual pathway. This led to developing a self-organising map of perceptual learning in the game of Go where 'expert' AIs could be compared with 'amateur' AIs, illustrating the different neural structures each AI had developed and what role each neuron played in perception, i.e. 'explainable AI'.
I currently research AI applied to finance and economics as well as teaching AI as part of my Criticality in Dynamical Systems postgraduate class. My earliest work in AI was implementing a shallow artificial neural network to simulate human-like perceptual expertise that mimicked the inferior temporal cortex of the human visual pathway. This led to developing a self-organising map of perceptual learning in the game of Go where 'expert' AIs could be compared with 'amateur' AIs, illustrating the different neural structures each AI had developed and what role each neuron played in perception, i.e. 'explainable AI'.
Artificial Intelligence in Complex Systems
Artificial intelligence takes many forms and in my work I've been interested in artificial neural networks as models of human perception, reinforcement learning and inverse reinforcement learning, as well as more general agent based models of complex systems. I work with AI in finance and economics as well as teaching AI as part of my "Criticality in Dynamical Systems" postgraduate class. My earliest work was implementing an artificial neural network to simulate human-like perceptual expertise that mimics the human visual system, the "ventral pathway". This led to developing a self-organising map of perceptual learning for the game of Go where 'expert' AIs could be compared with 'amateur' AIs, illustrating the different neural structures each AI had developed and what role each neuron plays in perception, i.e. 'explainable AI'.
AI and decision-making
AI can play three roles in economics: as a tool for data-analysis, as a model of human cognition and the emergence of collective behaviour, and as a model for how markets 'decide' what the price of an asset should be. I currently have PhD projects in information flow through markets as a form of computation and artificial neural networks used to model market dynamics. I have recently completed a federally funded grant on AI agents making decisions in housing markets.
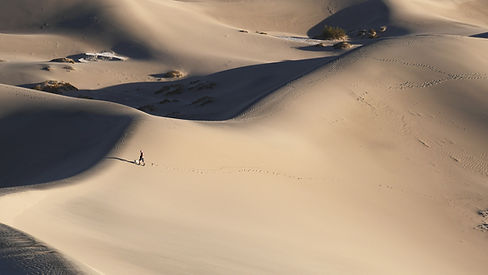
Publications
​
J Ruiz-Serra, MS Harré, (2023) Inverse Reinforcement Learning as the Algorithmic Basis for Theory of Mind: Current Methods and Open Problems, Algorithms 16 (2), 68
​
M.S. Harré (2022), What Can Game Theory Tell Us about an AI ‘Theory of Mind’? Games 13 (3), 46
​
M.S. Harré (2021) Information theory for agents in artificial intelligence, psychology, and economics Entropy 23(3), 310 https://www.mdpi.com/1099-4300/23/3/310
K.S. Glavatskiy, M. Prokopenko, A. Carro, P. Ormerod, M.S. Harré (2021) Explaining herding and volatility in the cyclical price dynamics of urban housing markets using a large scale agent-based model SN Business and Economics
​
B.P. Evans, K. Glavatskiy, M.S. Harré, M. Prokopenko (2021) The impact of social influence in Australian real-estate: market forecasting with a spatial agent-based model. Journal of Economic Interaction and Coordination.
​
Harré, M. (2018) Strategic Information Processing from Behavioural Data in Iterated Games. Entropy, 20(1), 1-12.
​
Harré, M. (2017) Utility, Revealed Preferences Theory, and Strategic Ambiguity in Iterated Games. Entropy, 19(5), 1-5
​
Wolpert, D., Harré, M., Olbrich, E., Bertschinger, N. & Jost, J. (2012). Hysteresis effects of changing the parameters of noncooperative games. Physical Review E. 85, 036102.
​
Wolpert, D., Jamison, J., Newth, D. & Harré, M. (2011). Strategic Choice of Preferences: The Persona Model, The B.E. Journal in Theoretical Economics, vol. 11, issue 1, article 18
​
​

AI and Perception
The US Air Force funded my research for three years during which I led a program on how perceptual patterns in complex environments such as Go could be learned by an AI that mimicked known psychological phenomena.
Publications
​
Harré, M. (2013) The Neural Circuitry of Expertise: Social Cognition and Perceptual Learning. Frontiers in Neurosciences: Neural Implementations of Expertise, 7:852.
Harré, M. (2013). From Amateur to Professional: A Neuro-Cognitive Model of Categories and Expert Development, Minds and Machines, Volume 23, Issue 4, pp 443-472
​
Harré, M., Bossomaier, T. & Snyder, A. (2012). The Perceptual Cues that Reshape Expert Reasoning, Nature: Scientific Reports, issue 2, article no. 502
​​
Harré, M. & Snyder, A. (2012). Intuitive Expertise and Perceptual Templates. Minds and Machines, p. 1-16
​
Bossomaier, T., Harré, M. & Thiruvarudchelvan, V. (2012). Seeing the Big Picture: Influence of Global Factors on Local Decisions. International Journal on Advances in Software, vol. 5, no. 1.
​
Harré, M., Bossomaier, T., Gillett, A. & Snyder, A. (2011). The Aggregate Complexity of Decisions in the Game of Go. Eur. Phys. J. B: Condensed Matter and Complex Systems, vol. 80, no. 4.
​
Harré, M., Bossomaier, T. & Snyder, A. (2011). The Development of Human Expertise in a Complex Environment. Minds and Machines, vol. 21, no. 3.
​